Wetlands provide critical ecosystem services across a range of environmental gradients and are at heightened risk of degradation from natural and anthropogenic drivers and continued development, especially in coastal regions. There is a growing need for high resolution, spatially and temporally, accurate habitat identification, and precise delineation of wetlands across a variety of stakeholder groups, including wetlands loss mitigation programs and especially the North Carolina Department of Transportation (NCDOT) Environmental Analysis Unit. Traditional wetland delineations are costly, time-intensive and can physically damage the systems that are being surveyed, while aerial surveys are relatively fast and unobtrusive.
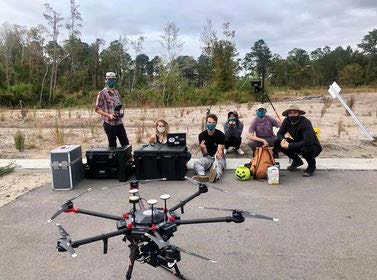
With funding from NCDOT, we acquired a DJI Matrice 600 Pro UAS outfitted with a Quanergy M8 LiDAR sensor in March 2020 and have conducted fieldwork at 9 sites in 4 counties in NC to test the performance of LiDAR-derived data in wetland classification. We test the comparative accuracies of a wetland prediction model fitted with UAS and airborne LiDAR terrain and topographic derivatives along with UAS-collected multispectral data at nine coastal sites using machine learning approaches. When mounted on low-flying UASs, LiDAR sensors can measure elevation data even underneath dense canopy cover characteristic of much of the Atlantic Plains forested wetlands. We show that the UAS hyperspatial LiDAR derivatives outperform the airborne LiDAR data in deriving high resolution and high classification accuracy rates, especially when trained with field-collected habitat data and supported by UAS-collected multispectral data. The hyperspatial resolution of LiDAR-derived topography models as well as vertical vegetation structure data (such as canopy height models) can fill wetlands mapping needs and increase accuracy and efficiency of detection and prediction of sensitive wetland ecosystems, thus ensuring more rapid and cost-efficient field assessments.